BLOG
Machine Learning: advantages and implementation for companies
Artificial intelligence, especially machine learning in companies, will become the most influential technology to transform industries and areas that are already in the focus of digitization from scratch.
With the proliferation of the Internet, the growing online presence and connected devices that generate a huge flood of digital information, companies are increasingly relying on algorithms to solve otherwise far-reaching problems with good guarantees of a solution. Data is the accelerator for our information society. Therefore, new technologies and machine learning techniques are the key to dealing with the new wealth of information in complex contexts.
Machine Learning – what is that?
But what is machine learning? Machine Learning as a branch of Artificial Intelligence (AI) uses algorithms and models that allow computers to perform tasks without explicit instructions. As a result, statements can be made based on human learning abilities, which help the system to automatically improve through this experience and provide accurate output based on new information. Put simply, Machine Learning finds answers to business-related problems. It is a data analysis process that uses algorithms to learn iteratively from existing data and help computers find hidden insights without being programmed to do so.
Machine learning can help companies to make data-driven decisions that result in savings and increased revenue. In addition, machine learning algorithms help eliminate risk and fraud, ensure secure processes and improve customer satisfaction. Surveys show that there are use cases for machine learning across all industries and all levels of companies. Artificial intelligence, and machine learning in particular, will not only have a significant impact on society over the next few years, but also and above all on the company’s own business areas.
Use cases of Machine Learning in companies
Machine Learning can be used to follow and understand customer conversations in the context of a product. It can even apply the algorithm to predict the functionality and features customers expect. In addition, a company can use machine learning to build better relationships with its customers. The machine learning algorithm can easily identify customer requests and send them to the appropriate team. It will accelerate the process of solving customers’ problems and provide them with quick answers.
When creating digital services, companies can use machine learning to enable customers to find products or information faster. A specific algorithm helps to ensure that customers receive relevant and high-quality information in a timely manner. In addition, the new technology helps customers to select products according to their needs and preferences.
Learning algorithms can be used in the financial industry to develop automated trading strategies. They can be used to identify patterns and make trading decisions based on data. The other potential applications are the creation of credit scoring mechanisms by searching for patterns of external, internal and economic factors that influence the financial performance of companies. These techniques can also be used to provide relevant automated investment advice to clients.
The speed with which Machine Learning identifies relevant data enables companies to take appropriate action at the right time. For example, Machine Learning optimises the best follow-up offer for a company’s customers. This enables the customer to see the right offer at the right time without an employee having to spend time on individual support or preparing offers. Machine Learning enables companies to analyse and interpret data on past behaviour or results. Based on the new and different data, better predictions about customer behavior can therefore be made.
Attacks on IT networks usually occur in real time without prior warning. To enable companies to maintain network security, any suspicious network behavior must be proactively identified before the intrusion leads to a full-scale security attack, data loss and outages. Machine learning algorithms help monitor network behavior for anomalies in real time so that proactive measures are automatically executed.
How companies implement Machine Learning
With the introduction of their cloud machine learning platforms by Google, Amazon and Microsoft Azure, the topic of artificial intelligence has gained in importance in recent years. There are various approaches to introducing machine learning in companies. The cost of data storage has fallen so dramatically that companies can access large amounts of data, hide the patterns of profitable business knowledge and use machine learning technologies to uncover them. Some organizations use cloud-based tools and third-party systems, while others use applications with built-in machine learning capabilities. Many algorithms and frameworks are accessible through open source channels. These pre-existing resources enable organizations to leverage machine learning without having to invest in the necessary infrastructure up front. The technology is already mature and companies can take advantage of the benefits.
These benefits can be applied to a wide range of use cases, especially when data is the focus of the service offering. The technology is rapidly replacing manual operations in the enterprise market segment, and both small and medium and large enterprises are well positioned to take advantage of machine learning solutions.
digital products that are capable of learning
Implementation of machine learning applications
Artificial intelligence (AI) and machine learning (machine learning) are the technologies with the greatest potential to completely reshape our future. Machine Learning provides us with tools to streamline business processes, improve decision-making and increase the accuracy of task solutions where humans are prone to error. All this, however, with the restriction that the required results do not have to be available immediately, because algorithms have to learn and the consumption of computing power can be immense. But not all machine learning applications and projects will be successful from the start. Many companies must first build the necessary end-to-end pipeline to support the continuous training of models.
The needs pyramid – prerequisites for the success of Data Science and Machine Learning
If you are not familiar with the Data Science pyramid of needs, Monica Rogati (former VP of Data at Jawbone and LinkedIn Data Scientist) describes the various requirements for success with Data Science and Machine Learning. Defining the basic technical and cultural requirements will probably take a long time. When it comes to the toolset, more comprehensive and “end-to-end” solutions such as Google’s Tensorflow and AWS Machine Learning will become attractive. However, it is likely that companies will have to compromise to adapt to their cloud strategy and use different tools until the market is mature.
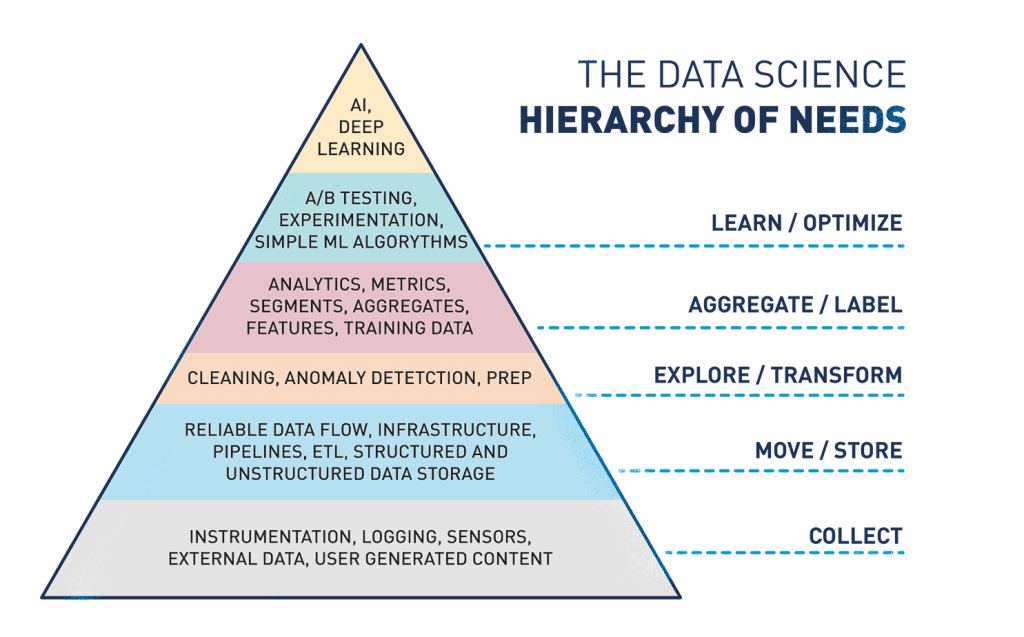
Source: Hackernoon https://hackernoon.com/the-ai-hierarchy-of-needs-18f111fcc007
For the implementation of machine learning, organizational issues must first be clarified, as well as the recruitment or training of specialists. In addition, there are still many measures that need to be taken to maximize investment in machine learning and to quickly integrate functionality into front-ends and digital services.
So how can apps and digital services be developed today that will enable us to use machine learning most efficiently tomorrow? Machine Learning can be taken into account at the design stage when new digital services are created, so that implementation is easier and more effective later. This is the approach:
1. creation of minimal machine learning models
The goal of every digital solution is to offer the user added value. The aim should therefore be to create added value for the user with as little effort (time and resources) as possible. If a Minimum Viable Product (MVP) cannot generate added value for users without Machine Learning, it is likely that it will not generate added value with it.
If for some reason an MVP cannot add value without using some kind of AI or machine learning capability (e.g. image recognition), then the use of cloud APIs that cover the most basic functionality should be considered. Services from AWS, Microsoft Azure or Google may not provide the highest accuracy for your use case, but they should be sufficient to validate the use case and justify a larger investment in building and training your own machine learning model. There are also open source projects that you can use to get started with common machine learning tasks. Ultimately, these services or open source projects can fully meet the requirements.
2. collecting data and events
Since data is the digital currency of machine learning, it is important to ensure that your digital product collects everything that could be relevant to the application. A prime example that should be collected in any digital product is user analysis. If you are able to collect information about how users interact with your application, what actions certain user segments perform, then this is data that could potentially be used in the future to improve usability through machine learning.
Any analysis tools you use should not only collect data, but most importantly, they should be exportable in a structured, clean and standardized format. These user analyses will not be of much use if they cannot be used to train machine learning models. Also make sure that you have the ability to create custom in-app events. This will allow you to collect all the correct metrics specific to your application.
Another fact to consider is the data processing and its legal framework of the analytics solution. Many analytics tools are SaaS models and allow you to access your data but store and process it in locations where the legal framework is not always clear or compliant. Many customers appreciate the fact that they have complete control over their data and that it is not used or stored in a third party environment, but in their own instance.
3. aggregate and structure existing data
Because many companies already have a treasure trove of data packed in databases and other silos of corporate IT (CRMs, ERPs, etc.). Often the data is also distributed across different systems and to obtain the most complete data set it may be necessary to obtain data from multiple sources and orchestrate it as needed. Often this is a media file or simply information that was not stored in the past but is relevant to the current use case.
When integrating data sources it is easy to aggregate and structure data from multiple sources. A popular example is the creation of a more complete view on the customer by combining data from multiple CRM or ERP systems. This way the data is almost ready for your machine learning pipeline. You can easily add attributes if additional labels or results are to be written from your machine learning model. This way you can customize data sets without making changes in your existing or company wide IT systems.
4. marking and export of data
Since adding labels and attributes to your records is easy, it is time to start with the actual labeling of the data. This can be done manually or automatically. No matter if the customer starts tagging his data, he always has the possibility to export all data via our REST API. Accessing the data through an API is essential as it allows you to automate the data export so that you can easily access the latest data set and use it to train new models.
5. use A/B tests when using new models
When deploying machine learning models, it is important that you do not assign 100% of your users to a new model, as errors or incorrect results would affect everyone. There are several approaches to implementing A/B testing, and ideally, it is then easy to revert to the old version or to roll out the new model to all your users.
6. start collecting further data as soon as possible when needed
Data is the lifeblood of machine learning and your data can determine the success or failure of your machine learning activities. When creating and training models, access to additional attributes can be the key to improving the model.
Implement machine learning capable products
So if you are planning to develop a digital product based on machine learning, start with an MVP and make sure you can deliver value to users. There’s nothing worse than making a huge investment in a digital product with – but also without – machine learning that offers no added value to users at all.